"AI for All" - navigating the path to inclusive innovation and equity
- Latera Tesfaye
- Dec 26, 2023
- 8 min read
Updated: Jan 3, 2024
Introduction
First, on my first blog, I added a button labeled "Go to Equitable AI Solutions" blog. However, whenever you try to click on it, the button shifts location, and you can never actually click on it. To me, this symbolizes the current issue related to equity, not just in AI but in many fields. It feels unreachable, a sort of out-of-reach mirage. Day by day, our actions exacerbate inequities, knowingly or unknowingly. I'm afraid that the equality touted in much of our research so far is just a collection of catchy phrases offering false hope. Unless we address economic disparities, true equity in any sector remains a dream. True, we might manage to satisfy basic needs for all, but for those in the highest ranking of wealth and capability, there is always another, more elusive need that emerges, a testament to the ever-evolving human desire. This hierarchy of needs, dictated by wealth and access, is a disservice to our collective humanity. In a world where some dine on possibilities while others starve for opportunities, the unmet need — basic or complex — is a glaring testament to the inequities that pervade our society. Equity is not just about meeting needs but about acknowledging and addressing the disparities that make the fulfillment of these needs an uneven landscape.
In the swiftly evolving landscape of technology, artificial intelligence (AI) emerges as a beacon of innovation, with the potential to fundamentally reshape industries, societies, and daily life. However, as AI technologies advance, a critical discourse is unfolding about the accessibility and equity of these developments. This blog delves into the current state of AI accessibility, the ethical challenges it poses, the importance of inclusivity, forces shaping AI equity, and the potential pathways towards a more equitable AI future.
Current State of AI Accessibility
AI’s groundbreaking advancements predominantly occur in high-income countries, leading to significant disparities in access and benefits. These disparities are not merely geographical but also economic and infrastructural. The cost of AI technologies, combined with the lack of necessary infrastructure in low- and middle-income countries, creates a chasm between those who can harness AI’s potential and those who are left behind. This divide not only limits the global reach of AI’s benefits but also raises concerns about the widening inequality. For example, 83% of the Ethiopian population will immediately have no access to AI solutions that require internet connectivity.
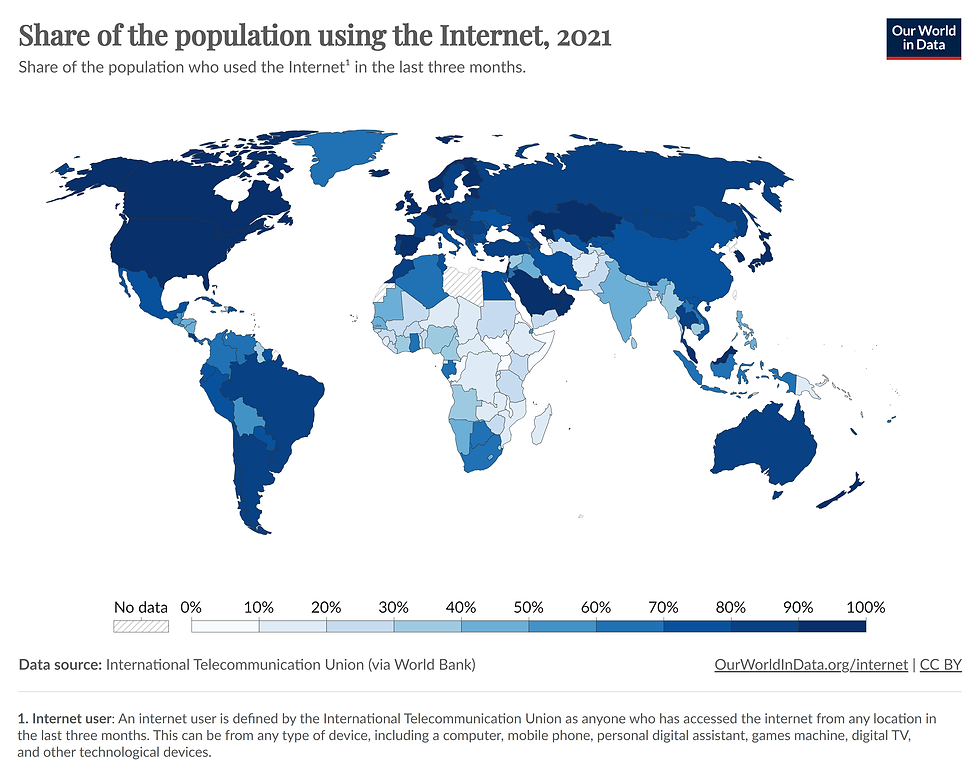
General ethical Considerations
The ethical landscape of AI is as complex as it is critical. Ethics in AI encompasses a range of issues, from privacy and security to fairness and bias. However, the relativity of ethical standards across different cultures and communities complicates the establishment of a universal ethical framework for AI. What is considered ethical in one society may not hold the same standing in another, leading to diverse and sometimes conflicting approaches to AI development. This ethical relativity poses a significant challenge to creating standardized, globally accepted guidelines for AI ethics.
Inclusivity in AI
At the heart of the discourse on AI accessibility and equity lies the principle of inclusivity. For AI to benefit humanity as a whole, it must account for the diverse needs and circumstances of different populations. This includes making AI technologies accessible and affordable, as well as ensuring that AI development considers a wide range of human experiences and conditions. Leading AI companies have a pivotal role to play in this regard. By integrating inclusivity into their missions and operations, they can drive a more equitable distribution of AI benefits. This involves not just making technologies accessible, but also actively working to include underrepresented communities in AI development and application.
Forces shaping AI equity
Force 1: Algorithmic/training data bias
Algorithmic bias represents a significant force shaping the equity of AI. This type of bias manifests when decision-making algorithms systematically marginalize certain groups, leading to adverse outcomes in critical sectors like healthcare, criminal justice, and finance. For instance, a study revealed a healthcare algorithm widely underestimating the healthcare needs of Black patients, resulting in significantly reduced care provisions. Such biases often stem from the underrepresentation of certain groups in the training data or the ingrained societal prejudices within the data. This issue is not only unjust but can also cause profound harm.
When considering training data bias, it can further perpetuate or even amplify these disparities, as these systems often learn and evolve based on the data they are fed, potentially reinforcing and spreading existing biases on a larger scale. If biased data is input into AI systems, they will inevitably produce biased results. For instance, consider a recruitment AI used by companies to screen job applicants. If this AI is trained on historical hiring data from a company that has a history of predominantly hiring men for certain roles, the AI might learn to favor male candidates over female candidates. As a result, even if equally or more qualified women apply for these positions, the AI could rank them lower, not because of their skills or potential, but simply because it reflects the bias present in its training data. This perpetuates the cycle of underrepresentation and can have long-lasting impacts on diversity and fairness in the workplace. Thus, the quality and diversity of the data used to train AI are crucial for ensuring its outputs are fair and unbiased.
In addition, if biased data is fed into generative AI models like GPT-4 or ChatGPT, the AI will likely generate biased results. For example, consider a scenario where a generative AI is trained on a dataset comprised predominantly of literary works by Western authors. If a user asks the AI for a global perspective on a specific topic, such as "the most influential literary works," the AI might generate a list heavily skewed towards Western literature, overlooking significant works from other regions. This bias doesn't stem from the AI's intentional preference but from the disproportionate representation in its training data. The AI's understanding and outputs reflect the biases present in the data it was trained on, demonstrating the critical need for diverse and balanced datasets in training generative AI to ensure equitable and representative results.
To this end, I recently conducted an experiment with DALL-E, generating ten images using various iterations of the prompt "Black American doctors treating white kids." Despite explicitly specifying that the children should be white in each variation, the AI consistently depicted at least one black children when paired with black doctors. This outcome highlights a potential limitation or bias in the AI's response to specific demographic prompts, underscoring the importance of understanding and addressing how these systems interpret and generate imagery based on the input they receive.
"White doctor with white kids" Vs "Black American doctor with white kids"
Force 2: Supply-side: who is impacted or benefitted more?
There is significant hype surrounding the notion that AI will facilitate extensive automation and augmentation, rendering repetitive tasks obsolete. For instance, such type of advancement promises to revolutionize tasks like code testing and creating generic functions, enabling programmers to concentrate on more creative aspects of their work. However, for low-paying jobs, which are predominantly occupied by people of color and other disadvantaged groups, perfect automation and augmentation could lead to job displacement. This raises important socioeconomic and ethical considerations, as the benefits of AI-driven efficiency might inadvertently exacerbate existing inequalities and economic disparities, necessitating thoughtful strategies to ensure a fair and inclusive transition in the workforce.
Potential Models for Change
To address the challenges of AI accessibility and equity, new models and approaches are needed. One potential pathway is reimagining AI as a public good, where the primary focus shifts from profit to maximizing social benefit. For example, ChatGPT. This could involve different funding models, such as philanthropic investments, public-private partnerships, and international collaborations. Additionally, policies and regulations can play a crucial role in guiding AI development towards greater equity and inclusivity. Keeping the balance between "reward for innovation", the cost of running AI-solutions, and the societal benefit is imperative.
Challenges and Pessimistic Outlook
Despite the potential pathways to a more equitable AI future, significant challenges remain. Skepticism and pessimism often stem from the historical difficulties in achieving global consensus on even simple issues. The same uncooperativeness that plague other areas of international cooperation are also present in the discourse on AI. The complexity of AI ethics, coupled with the rapid pace of technological development, which are concentrated in only certain parts of the world, adds another layer of difficulty to these challenges.
Conclusion
As AI continues to advance, the imperative to address its accessibility and equity issues becomes increasingly urgent. While the path forward is fraught with challenges, it is also filled with potential. By committing to inclusivity, exploring new models for AI as a public good, and fostering international collaboration, we can work towards a future where AI benefits all of humanity. The journey towards greater AI accessibility and equity is not just a technological challenge but a moral imperative, requiring concerted effort from companies, policymakers, and individuals worldwide.
Finally, one aspect I don't entirely agree with is the forceful adjustment of our model to conform to a given political and social ideologies, regardless of whether they are right or wrong. My point is that we need to be selective about the data we use in the training. Allow me to share my experience with ChatGPT around its initial release. I wanted to create a scenario around backward racist stereotypes to see how ChatGPT responds. The scenario was: four Black American scientists conducted a study on the relationship between the number of Black Americans living in a certain area and the crime rate in that area. I indicated the researchers had done everything they could to make their research absolutely perfect (like controlling for all confounders, such as the economy, existing crime rates, etc.), and then they found, "the more Black Americans in a certain area, the higher the crime rate." I asked ChatGPT whether it should find their findings credible or not. ChatGPT said, "Yes, it's credible." Next, I maintained all other scenarios the same but changed the Black researchers to white researchers. Surprisingly, the answer is now, "No, it is not credible." This raises many concerns, which I won't delve into here. However, one of the questions ChatGPT asked was why all the researchers were white. It suggested there might be systematic biases or hidden objectives behind the research. I completely agree, but this might also apply to Black researchers. I think the initial response of ChatGPT might be related to the simple fact that the researchers are Black, as if it was trained to do so. Navigating through such backward racist stereotypes in models starts by carefully refining our training datasets, rather than just training the model on everything and then trying to limit the model's parameters to give us an answer that is deemed correct.
Distributing AI solutions widely is a commendable step towards achieving equity in AI, but it is far from a complete solution. True equity and accessibility in AI are not just about spreading just the solutions (outputs/products); they are fundamentally about enabling a diverse range of entities to create and sustain their own AI models. This approach ensures that the benefits of AI aren't just confined to those with the most resources. The crux of democratizing AI lies in ensuring that other companies, especially those with fewer resources, can develop underlying models that drive these solutions. These models need to be efficient and affordable, capable of running on less complex and less expensive infrastructure while still delivering consistent, high-quality results. By lowering the barriers to entry, smaller companies and organizations from underrepresented regions and sectors can contribute to the AI landscape, fostering innovation and tailoring solutions to a wider range of needs and contexts.
Comments